Causal Machine Learning
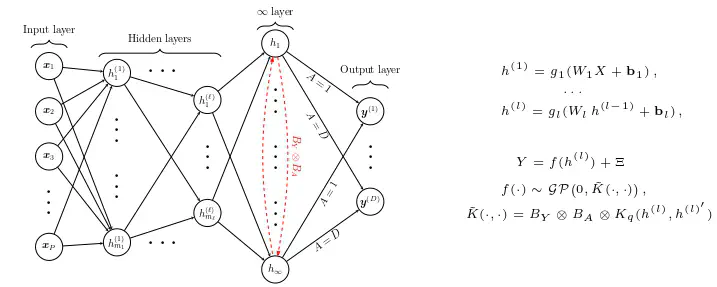
In the recent years, there has been a surging interest in the use of Statistical/Machine Learning tools for causal inference - and the other way around as well (“Causality for Machine Learning”). These tools leverage large datasets and usually deliver improved performance, provided that they are properly adjusted to be used in causal settings.
The broad idea of this project is to design and develop ML methods for estimating causal effects for highly personalized policy-making (e.g., heterogeneous treatment effects, spillover effets, etc.).